“Rhizomatiks Beyond Perception” is a solo exhibition themed around “AI and Generative Art,” held in conjunction with the expansion and relocation of KOTARO NUKAGA (Tennoz). For this exhibition, Rhizomatiks developed a new AI model that was trained from scratch using only Rhizomatiks’ original images, without relying on any existing foundation models. Through this exhibition, the question is raised: “What is the value of generated images?” in an era where anyone can use AI to create images.
‘Rhizomatiks Beyond Perception’
Date: June 29 (Sat) – October 14 (Mon), 2024
11:00 – 18:00 (Tue – Sat)
*Closed on Sun, Mon and Public Holidays
*Summer Holidays August 11(Sun) – 19(Mon)
*Opened on October 13(Sun)and 14(Mon)
VENUE KOTARO NUKAGA (Tennoz)
TERRADA Art Complex II 1F, 1-32-8 Higashi-Shinagawa, Shinagawa-ku, Tokyo, 140-0002 Japan
*In this exhibition, the AI model (priced at 5.5 million yen + tax / 5 editions) and acrylic panels generated by this model (priced at 300,000 yen + tax / 30 editions) are available for purchase.
*For this exhibition, collectors who obtain the model receive a usage license for the model. Based on this license, collectors can use the model, which has been trained solely on images created by Rhizomatiks, to generate images for both commercial and non-commercial purposes. Additionally, they can further train the model using images they have created themselves or images for which they have obtained authorization to use for training the model.
Technical Information
Rhizomatiks has created the “Beyond Perception Model,” an image generation AI trained exclusively on Rhizomatiks’ visual works. This page explains the development process of the “Beyond Perception Model.”
Dataset Creation
1. Material Collection
We collected video materials created in-house, for which we hold the copyright. Combining previously created videos and newly produced videos specifically for this exhibition, a total of 108 video materials were prepared. Each video material ranges from 30 seconds to 4 minutes in length. These were converted into still images on a frame-by-frame basis, resulting in approximately 170,000 still images being prepared as training data.
2. Annotation
Labeling (annotation) was performed for each image. However, as most of the videos consisted of abstract graphics without specific objects, it was determined that assigning appropriate captions to each video would be difficult. Instead, a unique ID was assigned to each video, and a dataset of image-ID pairs was created.
For example, images 1-1000 converted from video 1 were assigned ID1, images 1001-2000 converted from video 2 were assigned ID2, and so on, as classification labels.
3. Model Characteristics
As a result of this approach, the Beyond Perception Model developed in this study was not trained as a model that generates images from text prompts, but as a “class-conditioned” model that generates images by combining unique IDs assigned to each video and their weight. Consequently, this model does not utilize text encoders such as CLIP Text Encoder.
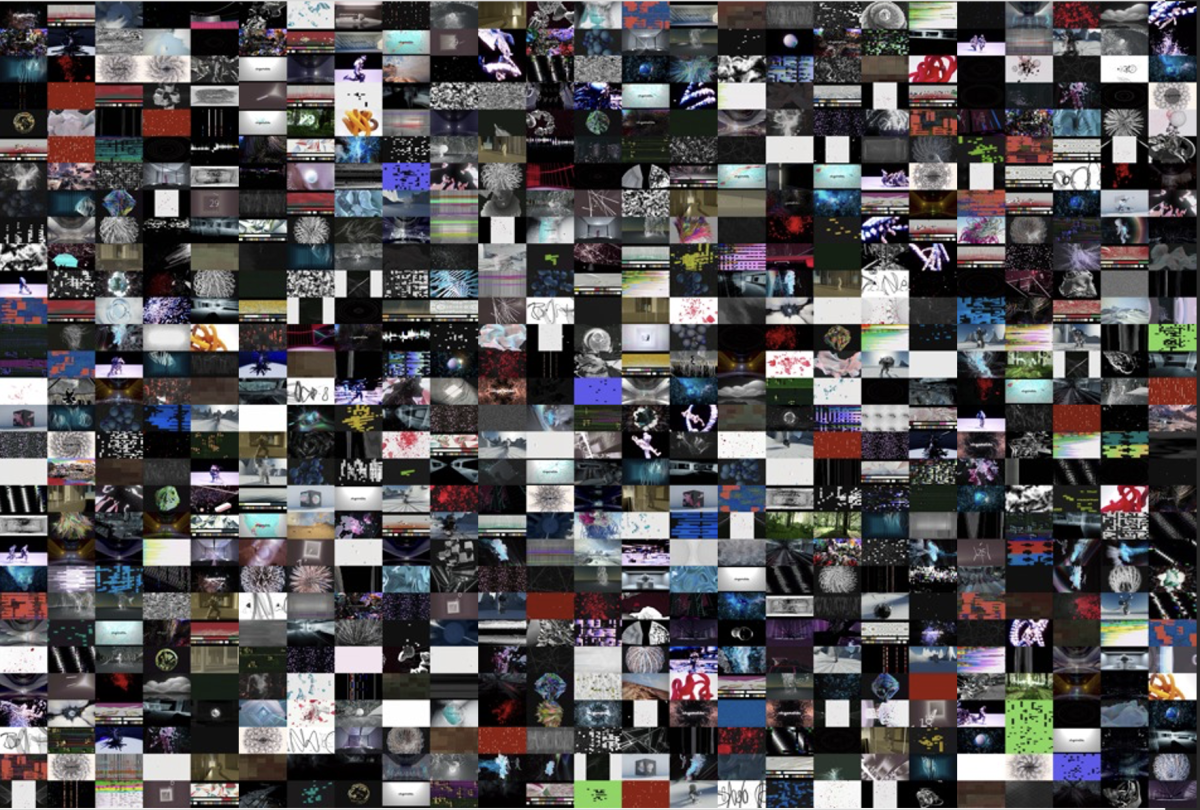
Trained images ( selected)
Determination of Model Structure
The present model was designed as a unique latent diffusion model with a small number of parameters, incompatible with existing image generation AI. This design was based on two considerations: full-scratch training using only proprietary data, and the ability to operate image generation processes on small embedded devices such as Jetson.
The base algorithm adopts a latent diffusion model [2], which is an evolution of the diffusion model [1] that has been used in many image generation AIs that have gained attention since 2022. A diffusion model learns the reverse diffusion process of gradually removing noise from a Gaussian noise image. During training, noise is incrementally added to the training images, eventually transforming them into pure Gaussian noise images.
As mentioned earlier, the input for generation in the “Beyond Perception Model” is not a text prompt, but a combination of unique video IDs and weight values. By directly specifying these, it is possible to generate images that mix components of the training data videos. Furthermore, by specifying multiple video unique IDs and weight individually for each generation step, more diverse outputs can be obtained. For example, in the current exhibition, all image generation is performed in 50 steps, with specifications such as ID 8 at weight 0.8 for steps 1-20, ID 35 at weight 1.2 for steps 10-30, and ID 78 at weight 1.0 for steps 10-50.
In situations with limited overall training data, as in this case, such class-conditioned models can achieve better training results.
The training of this model consists of two modules: U-Net and VAE.
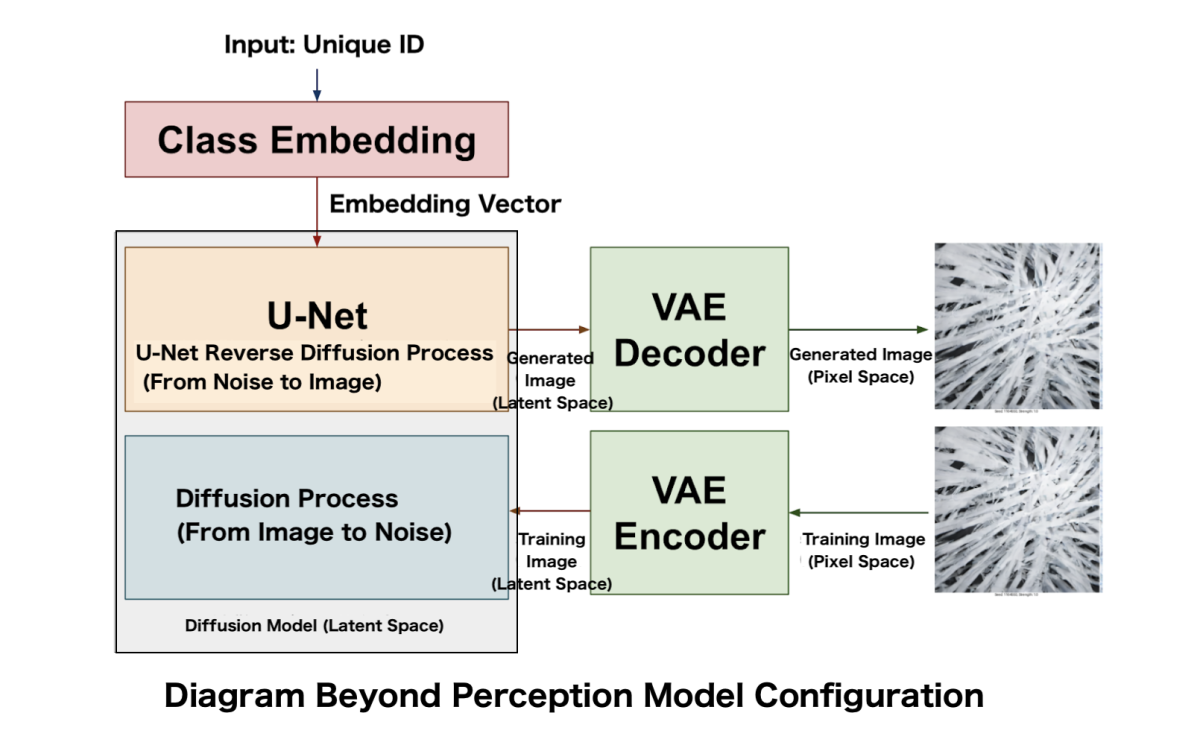
U-Net is the core module for image generation, generating small images from noisy images. Many elements that compose the image, such as composition and subject matter, are determined in U-Net.
VAE is a module for mutual conversion between pixel representation of images and a more compact latent representation. During generation, it performs conversion from latent space to pixel space (Decoder). During training, it performs the reverse conversion from pixel space to latent space (Encoder).
Training
The training itself was conducted in the order of VAE → U-Net.
VAE training also utilized the Rhizomatiks dataset of 170,000 images explained in Chapter 1, performing full-scratch training. The purpose of VAE training is to obtain a latent representation by encoding (compressing) images, and the training process aims to minimize the error between the decoded image and the original image when the image is encoded (compressed/downscaled) and then decoded (expanded/upscaled). As a result, the model obtains an encoder-decoder that can efficiently compress the dataset images.
In VAE training, LPIPS [3] is often adopted as perceptual loss. However, as LPIPS is also a neural network and trained with data of unclear licensing, LPIPS loss was not used. Instead, VAE training was conducted using Wavelet loss [4], which can be solely mathematically defined and does not raise concerns about the licensing of training data.
U-Net was also trained from scratch using the “dataset of 170,000 image-ID pairs created from videos independently produced and copyrighted by Rhizomatiks”.
U-Net training began at a low resolution of 256×256 pixels and gradually transitioned to higher resolutions, eventually allowing for image generation at 896×512 pixels.Throughout this process, multiple training sessions were conducted with fine-tuning of the parameters. The final “Beyond Perception Model” was completed by integrating a total of 8 training processes.
Reference
[1]
“Denoising Diffusion Probabilistic Models”https://arxiv.org/abs/2006.11239
[2]
“High-Resolution Image Synthesis with Latent Diffusion Models”https://arxiv.org/abs/2112.10752
[3]
“The Unreasonable Effectiveness of Deep Features as a Perceptual Metric”https://arxiv.org/abs/1801.03924
[4]
“Wavelet Loss Function for Auto-Encoder”https://ieeexplore.ieee.org/document/9351990